Evaluation methods
LLM-as-a-judge
In the "Evaluation methods" section of the New Job form, activate the "AI scoring" section and fill in details.
You can describe in plain english the properties you are trying to evaluate and grade. You can create as many scoring metrics as you want.
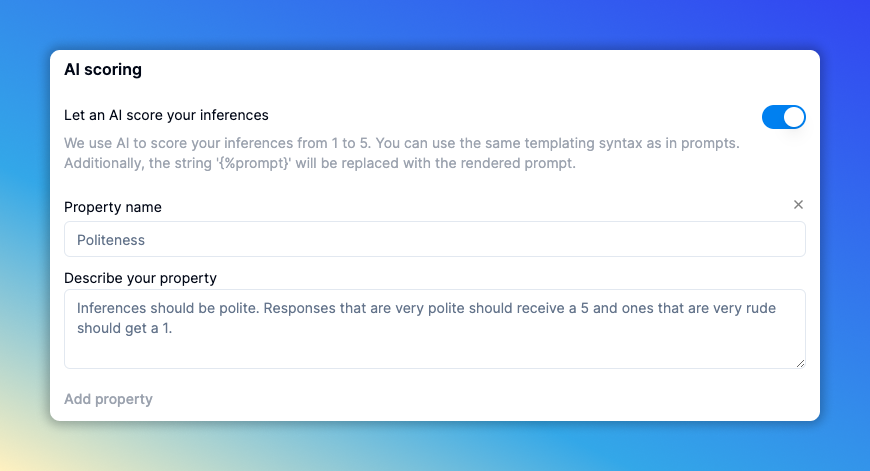
Templating
The description of the property can be templated using the same templating as model prompts. Double curly brackets {{value}}
will interpolate with the value
field from the input dataset.
Additionally, {%prompt}
will interpolate to the fully rendered input prompt.
Tips to maximize score quality
Here are some tips to get the best possible results out of our scoring model.
Unidimensional properties
Make sure to break down the properties you want to evaluate into their most elementary components. The more focused and straightforward the property, the easier it will be for the scoring model to reason about it.
For example, instead of defining a broad property as such
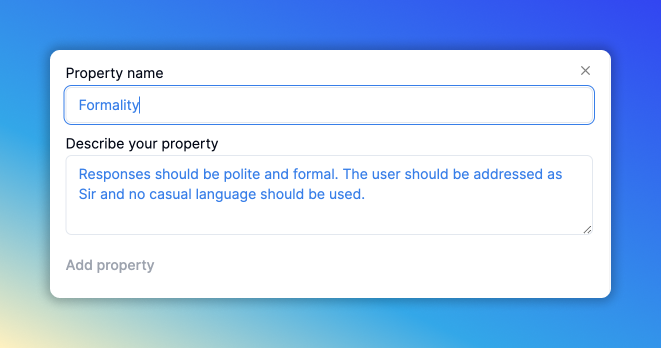
prefer breaking it down into three properties
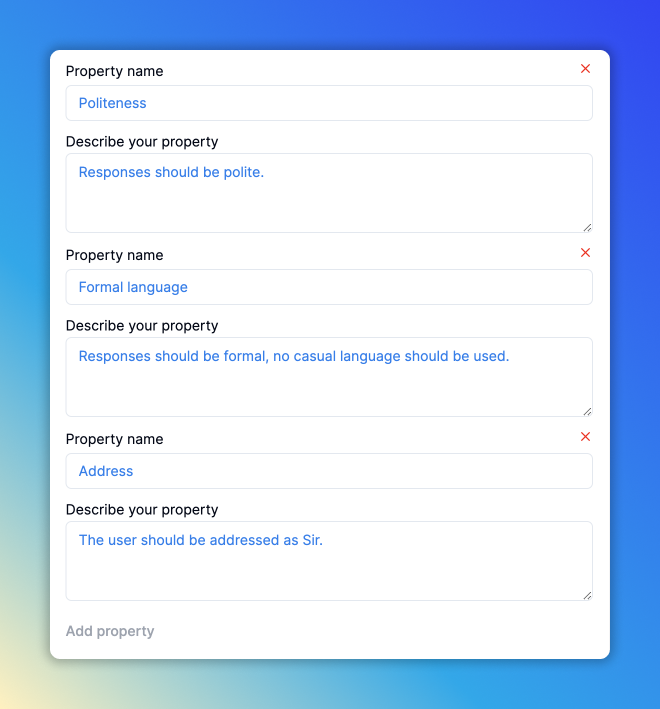
Add a rubric
We have observed that using a rubric yields more accurate score. Try to provide specific criteria for each score. You can use the 1 to 5 range to design your own scoring range.
For example:
The score describes how well justified the response is based on the context in the prompt. The following is a grading rubric to use:
1. The answer is incorrect OR the reasoning behind the answer doesn't reference the context at all.
2. The answer is incorrect, OR it uses an external statistic, book, or other reference as a key element in its reasoning.
3. The answer may or may not be correct. It may make reference to external facts, but only if they are fairly common knowledge.
4. The answer is correct and was derived from the context using almost no external knowledge.
5. The answer is correct. Either the answer is directly in the context in a way that requires no derivation, or the derivation uses only rigorous reasoning like correctly applied math or spatial reasoning.
Now, for the response you are grading, the context and question are:
<context>{{context}}</context>
<question>{{question}}</question>
Unsupervised metrics
Airtrain offers a number of standard metrics that can be evaluated without labeled references. The following metrics are available:
- Length – simply the number of characters in the inference
- Compression – Ratio of input length to output length with lengths measured in characters (paper)
- Extractive Fragment Density – How much of the output is formed by pulling fragments from the input. (paper)
If you would like other metrics implemented, reach out to us on Slack.
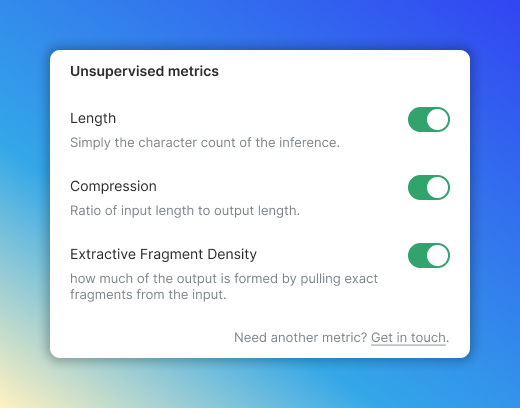
JSON schema validation
Airtrain enables validation of JSON payload. Your schema should be defined using the JSON Schema specification.
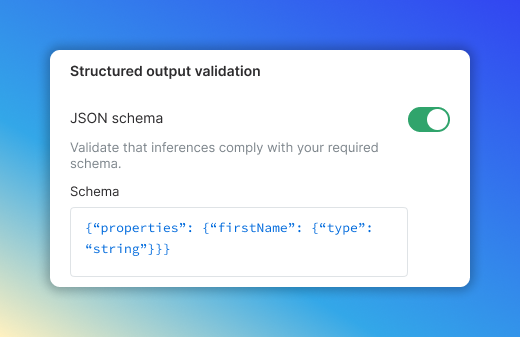
You can use the same templating language here as for prompt or response templates.
Reference-based metrics
In general, generative AI produces outputs where there are many diverse inferences that are all equally valid (ex: there are many possible responses to "tell me a story about a dragon" which would be valid but differ wildly). However, for other use-cases the correct answer can be known with a high degree of accuracy. As an example, if the prompt was "Which of these colors is in the middle of a rainbow: red, yellow, or violet? Respond with the color and no other text.", we would expect the response to be "yellow". For these cases, it's appropriate to use reference based metrics.
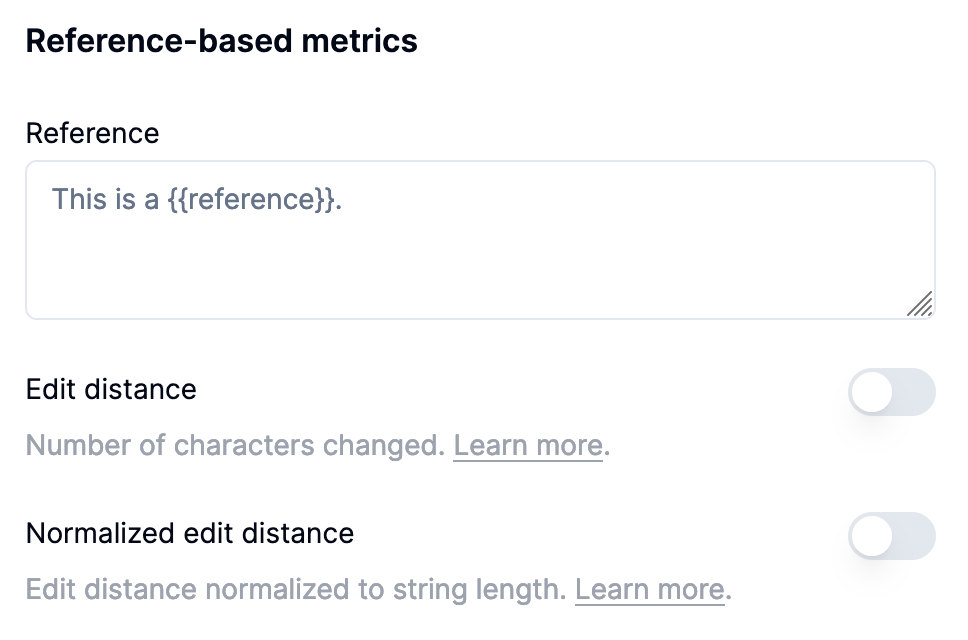
To use reference-based metrics in Airtrain, you need to populate the "Reference" field with a template for what the reference should look like. You can use the same templating rules as for prompt or response templates. The available fields to populate the template will be pulled from the jsonl or csv file you uploaded. For example, if your source data has a field called "answer", and the model is supposed to output the answer by saying "[ANSWER]: " followed by the answer, your reference template might look like this:
[ANSWER]: {{answer}}
Once you have provided a reference, you can toggle various metrics to compute with it.
Metrics
Edit distance
This is the Levenshtein distance between the reference text and the actual model's response. Roughly, this corresponds to the number of characters you would need to insert, remove, or replace to convert from the model's response to the reference.
Normalized edit distance
This is the Levenshtein edit distance divided by the max of the number of characters in the response or reference. This can be useful if you care more about the relative frequency of the number of errors in different texts (i.e. errors per character) rather than the absolute number of errors.
Updated 5 months ago